Although the potential of virtual treatment planner network (HieVTPN) in automating radiotherapy (RT) treatment planning has been demonstrated, its poor scalability caused by an almost linear growth of network size with the number of treatment planning parameters (TPPs) is a bottleneck, preventing its application in complicate, but clinically relevant planning problems. Motivated by the decision-making process of a human planner, this study proposes a hierarchical framework. The proposed hierarchical VTPN (HieVTPN) consists of Structure-Net, Parameter-Net, and Action-Net. When interacting with a TPS, these networks are employed in a sequential order to decide the structure, TPP, and the specific adjustment manner, respectively. We developed a hierarchical deep reinforcement learning (DRL) scheme to simultaneously train the three networks. We evaluate the effectiveness of he proposed framework using the prostate cancer intensity modulated RT (IMRT) planning and stereotactic body RT (SBRT) planning as testbeds. The performance was compared to VTPN of a parallel architecture, and the human plans submitted for competition in the 2016 American Association of Medical Dosimetrist (AAMD)/Radiosurgery Society (RSS) Plan Study. We analyzed scalability of the HieVTPN and VTPN. Numerical experiments were performed to understand the rationale of the decision-making behaviors of HieVTPN. Both HieVTPNs for IMRT and SBRT were trained successfully. For IMRT, HieVTPN achieved an average plan score of 8.62 (±0.83) on testing patients, with 9 being the maximum, which is comparable to 8.45 (±0.48)of VTPN. For SBRT, HieVTPN achieved an average testing score of 139.07 compared to 132.21 of the human plans submitted for competition. Different from VTPN, the network size of HieVTPN is almost independent of the number of TPPs. Also the decision-making behaviors of HieVTPN generally agreed with the human experience. With the scalability and explainability, the HieVTPN is more favorable than the previous framework while handling planning problems involving a large number of TPPs.© 2021 Institute of Physics and Engineering in Medicine.
A hierarchical deep reinforcement learning framework for intelligent automatic treatment planning of prostate cancer intensity modulated radiation therapy.
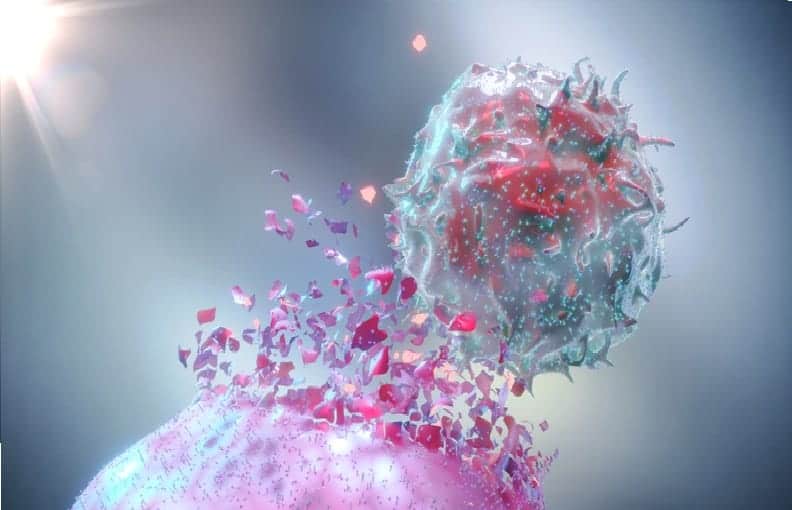