Snoring is a prevalent phenomenon. It may be benign, but can also be a symptom of obstructive sleep apnea (OSA) a prevalent sleep disorder. Accurate detection of snoring may help with screening and diagnosis of OSA.
We introduce a snore detection algorithm based on the combination of a convolutional neural network (CNN) and a recurrent neural network (RNN). We obtained audio recordings of 38 subjects referred to a clinical center for a sleep study. All subjects were recorded by a total of 5 microphones placed at strategic positions around the bed. The CNN was used to extract features from the sound spectrogram, while the RNN was used to process the sequential CNN output and to classify the audio events to snore and non-snore events. We also addressed the impact of microphone placement on the performance of the algorithm.
The algorithm achieved an accuracy of 95.3 ± 0.5%, a sensitivity of 92.2 ± 0.9%, and a specificity of 97.7 ± 0.4% over all microphones in snore detection on our data set including 18412 sound events. The best accuracy (95.9%) was observed from the microphone placed about 70 cm above the subject’s head and the worst (94.4%) was observed from the microphone placed about 130 cm above the subject’s head.
Our results suggest that our method detects snore events from audio recordings with high accuracy and that microphone placement does not have a major impact on detection performance.
Copyright © 2020. Published by Elsevier B.V.
Audio-based snore detection using deep neural networks.
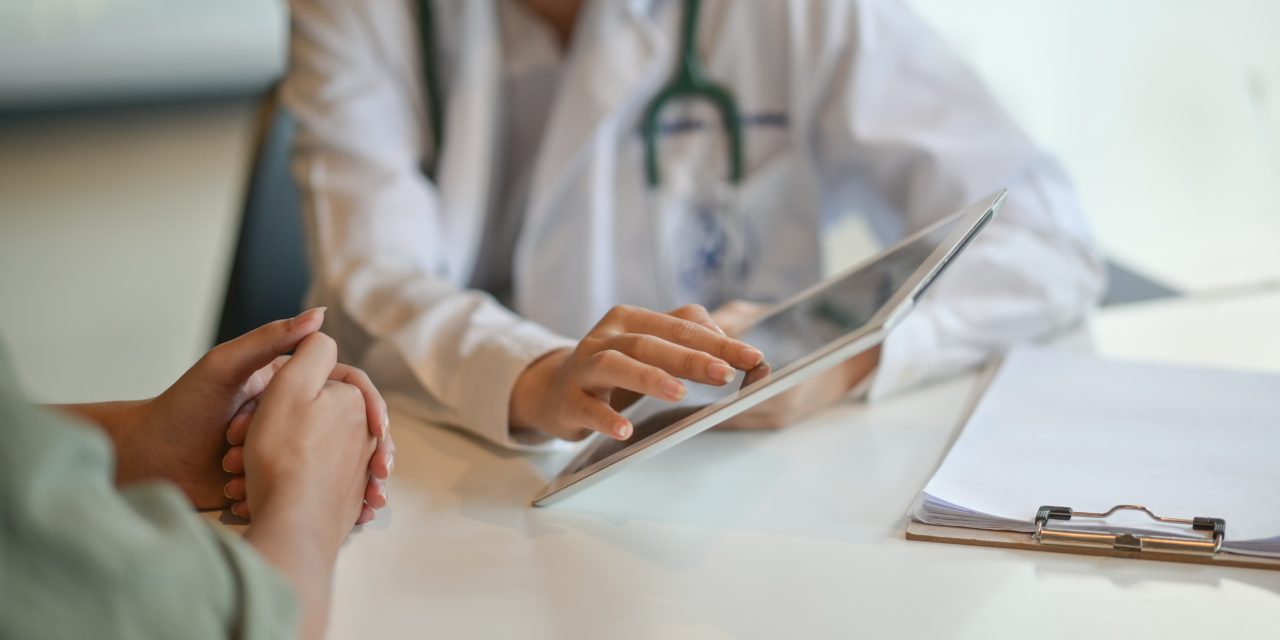