Pneumonia is a disease that affects the lungs, making breathing difficult. Nowadays, pneumonia is the disease that kills the most children under the age of five in the world, and if no action is taken, pneumonia is estimated to kill 11 million children by the year 2030. Knowing that rapid and accurate diagnosis of pneumonia is a significant factor in reducing mortality, acceleration, or automation of the diagnostic process is highly desirable. The use of computational methods can decrease specialists’ workload and even offer a second opinion, increasing the number of accurate diagnostics.
This work proposes a method for constructing a specific convolutional neural network architecture to detect pneumonia and classify viral and bacterial types using Bayesian optimization from pre-trained networks.
The results obtained are promising, in the order of 0.964 accuracy for pneumonia detection and 0.957 accuracy for pneumonia type classification.
This research demonstrated the efficiency of CNN architecture estimation for detecting and diagnosing pneumonia using Bayesian optimization. The proposed network proved to have promising results, despite not using common preprocessing techniques such as histogram equalization and lung segmentation. This fact shows that the proposed method provides efficient and high-performance neural networks since image preprocessing is unnecessary.
Copyright © 2021 Elsevier B.V. All rights reserved.
Bayesian convolutional neural network estimation for pediatric pneumonia detection and diagnosis.
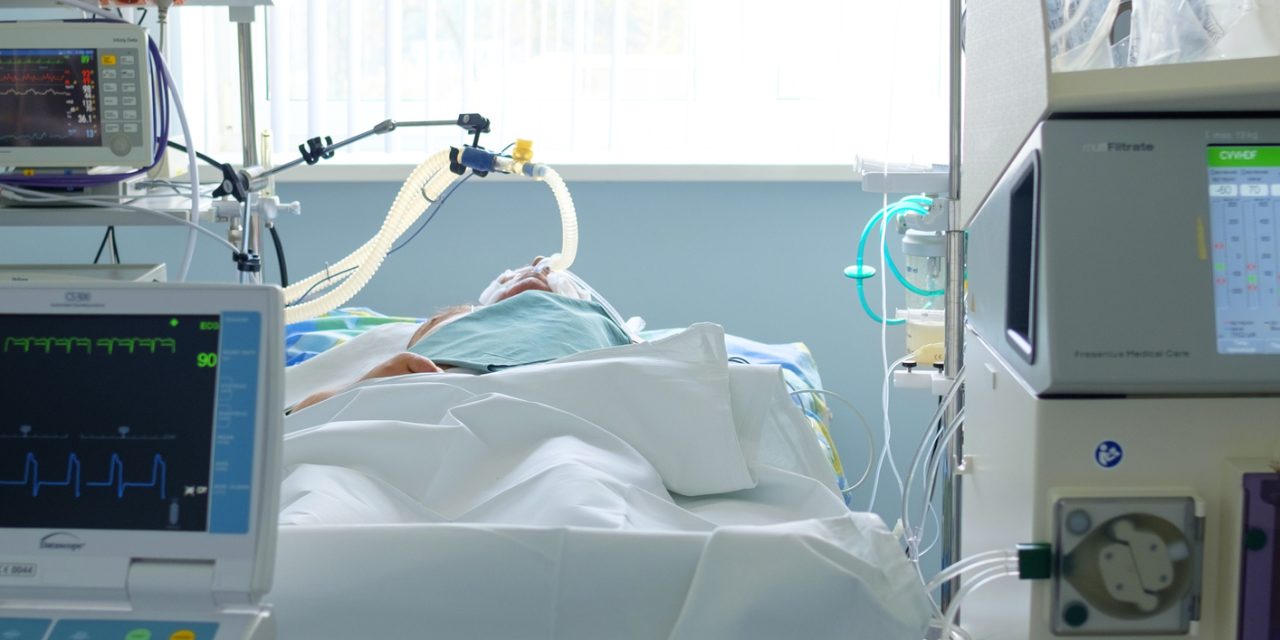