A subset of primary central nervous system lymphomas (PCNSL) are difficult to distinguish from glioblastoma multiforme (GBM) on magnetic resonance imaging (MRI). We developed a convolutional neural network (CNN) to distinguish these tumors on contrast-enhanced T-weighted images. Preoperative brain tumor MRIs were retrospectively collected among 320 patients with either GBM (n = 160) and PCNSL (n = 160) from two academic institutions. The individual images from these MRIs consisted of a training set (n = 1894 GBM and 1245 PCNSL), a validation set (n = 339 GBM; 202 PCNSL), and a testing set (99 GBM and 108 PCNSL). Three CNNs using the EfficientNetB4 architecture were evaluated. To increase the size of the training set and minimize overfitting, random flips and changes to color were performed on the training set. Our transfer learning approach (with image augmentation and 292 epochs) yielded an AUC of 0.94 (95% CI: 0.91-0.97) for GBM and an AUC of 0.95 (95% CI: 0.92-0.98) for PCNL. In the second case (not augmented and 137 epochs), the images were augmented prior to training. The area under the curve for GBM was 0.92 (95% CI: 0.88-0.96) for GBM and an AUC of 0.94 (95% CI: 0.91-0.97) for PCNSL. For the last case (augmented, Gaussian noise and 238 epochs) the AUC for GBM was 0.93 (95% CI: 0.89-0.96) and an AUC 0.93 (95% CI = 0.89-0.96) for PCNSL. Even with a relatively small dataset, our transfer learning approach demonstrated CNNs may provide accurate diagnostic information to assist radiologists in distinguishing PCNSL and GBM.© 2021. The Author(s).
Classification of glioblastoma versus primary central nervous system lymphoma using convolutional neural networks.
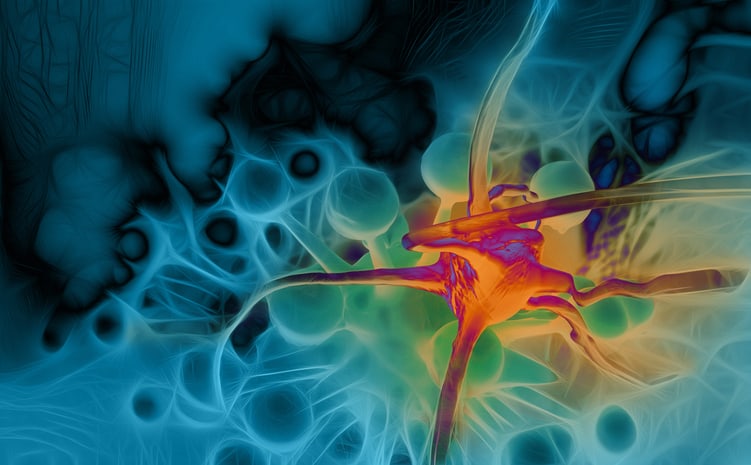