Diagnosis of esophageal cancer or precursor lesions by endoscopic imaging depends on endoscopist expertise and is inevitably subject to interobserver variability. Studies on computer-aided diagnosis (CAD) using deep-learning or machine-learning are on the rise. However, studies with small sample sizes are limited by inadequate statistical strength. Here, we used a meta-analysis to evaluate the diagnostic test accuracy (DTA) of CAD algorithms of esophageal cancers or neoplasms using endoscopic images.
Core databases were searched for studies based on endoscopic imaging using CAD algorithms for the diagnosis of esophageal cancer or neoplasms and presenting data on diagnostic performance, and a systematic review and DTA meta-analysis were performed.
Overall, 21 and 19 studies were included in the systematic review and DTA meta-analysis, respectively. The pooled area under the curve, sensitivity, specificity, and diagnostic odds ratio of CAD algorithms for the diagnosis of esophageal cancer or neoplasms in the image-based analysis were .97 (95% confidence interval [CI], .95-.99), .94 (95% CI, .89-.96), .88 (95% CI, .76-.94), and 108 (95% CI, 43-273), respectively. Meta-regression showed no heterogeneity, and no publication bias was detected. The pooled area under the curve, sensitivity, specificity, and diagnostic odds ratio of CAD algorithms for the diagnosis of esophageal cancer invasion depth were .96 (95% CI, .86-.99), .90 (95% CI, .88-.92), .88 (95% CI, .83-.91), and 138 (95% CI, 12-1,569), respectively.
CAD algorithms showed high accuracy for the automatic endoscopic diagnosis of esophageal cancer and neoplasms. The limitation of lacking performance in external-validation and clinical application should be overcome.
Copyright © 2020 American Society for Gastrointestinal Endoscopy. Published by Elsevier Inc. All rights reserved.
Computer-aided diagnosis of esophageal cancer and neoplasms in endoscopic images: a systematic review and meta-analysis of diagnostic test accuracy.
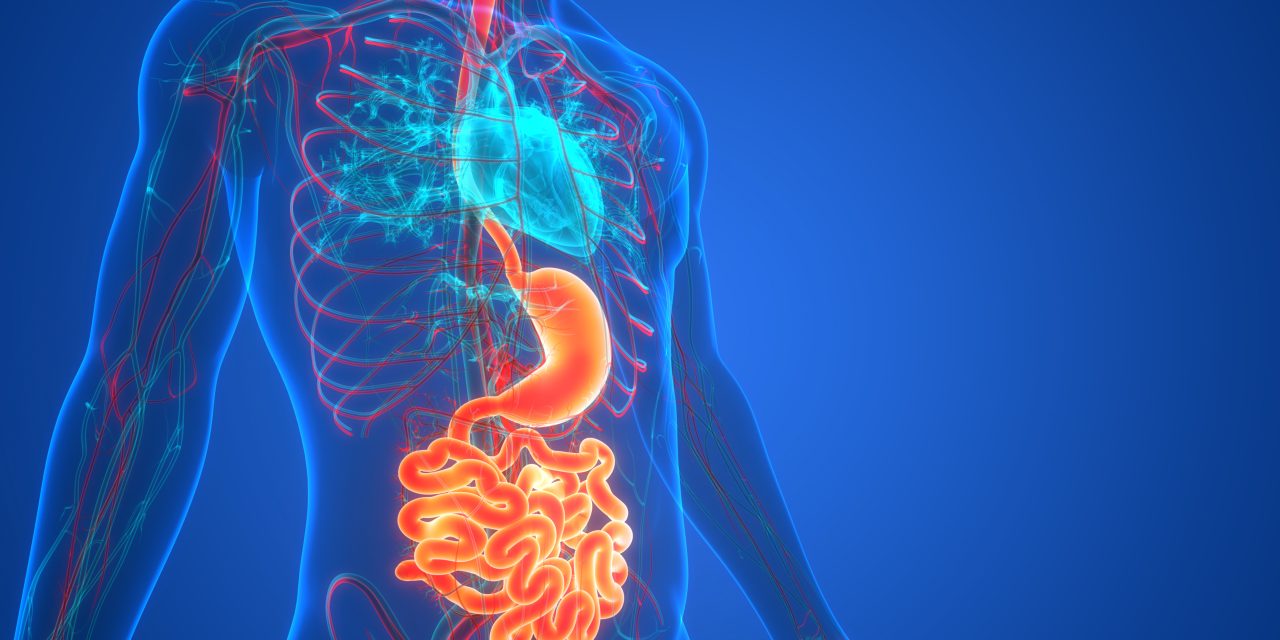