Adenocarcinoma (ADC) is the most common histological subtype of lung cancers in non-small cell lung cancer (NSCLC) in which ground glass opacifications (GGOs) found on computed tomography (CT) scans are the most common lesions. However, the presence of a micropapillary or a solid component is identified as an independent predictor of prognosis, suggesting a more extensive resection. The purpose of our study is to explore imaging phenotyping using a method combining radiomics with deep learning (RDL) to predict high-grade patterns within lung ADC.
Included in this study were 111 patients differentiated as having GGOs and pathologically confirmed ADC. Four different groups of methods were compared to classify the GGOs for the prediction of the pathological subtypes of high-grade lung ADCs in definitive hematoxylin and eosin stain, including radiomics with gray-level features, radiomics with textural features, deep learning method, and the RDL.
We evaluated the performance of different models on 111 NSCLC patients using 4-fold cross-validation. The proposed RDL has achieved an overall accuracy of 0.913, which significantly outperforms the other methods (p < 0.01, analysis of variation, ANOVA). In addition, we also verified the generality and practical effectiveness of these models on an independent validation dataset of 28 patients. The results showed that our RDL framework with an accuracy of 0.966 significantly surpassed other methods.
High-grade lung ADC based on histologic pattern spectrum in GGO lesions might be predicted by the framework combining radiomics with deep learning, which reveals advantage over radiomics alone.
Copyright © 2020 Elsevier B.V. All rights reserved.
Deep learning combined with radiomics may optimize the prediction in differentiating high-grade lung adenocarcinomas in ground glass opacity lesions on CT scans.
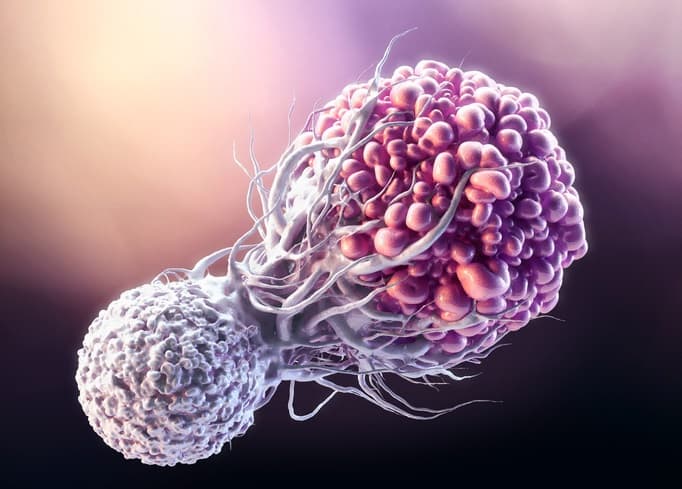