Ischemic heart disease (IHD), in its chronic stable form, is a subtle pathology due to its silent behavior before developing in unstable angina, myocardial infarction or sudden cardiac death. The clinical assessment is based on typical symptoms and finally confirmed, invasively, by coronary angiography. Recently, heart rate variability (HRV) analysis as well as some machine learning algorithms like Artificial Neural Networks (ANNs) were used to identify cardiovascular arrhythmias and, only in few cases, to classify IHD segments in a limited number of subjects. The goal of this study was the identification of the ANN structure and the HRV parameters producing the best performance to identify IHD patients in a non-invasive way, validating the results on a large sample of subjects. Moreover, we examined the influence of a clinical non-invasive parameter, the left ventricular ejection fraction (LVEF), on the classification performance.
To this aim, we extracted several linear and non-linear parameters from 24h RR signal, considering both normal and ectopic beats (Heart Rate Total Variability), of 251 normal and 245 IHD subjects, matched by age and gender. ANNs using several different combinations of these parameters together with age and gender were tested. For each ANN, we varied the number of hidden neurons from 2 to 7 and simulated 100 times changing randomly training and test dataset.
The HRTV parameters showed significant greater variability in IHD than in normal subjects. The ANN applied to meanRR, LF, LF/HF, Beta exponent, SD2 together with age and gender reached a maximum accuracy of 71.8% and, by adding as input LVEF, an accuracy of 79.8%.
The study provides a deep insight into how a combination of some HRTV parameters and LVEF could be exploited to reliably detect the presence of subjects affected by IHD.
© 2020 Institute of Physics and Engineering in Medicine.
Detection of subjects with ischemic heart disease by using machine learning technique based on heart rate total variability parameters.
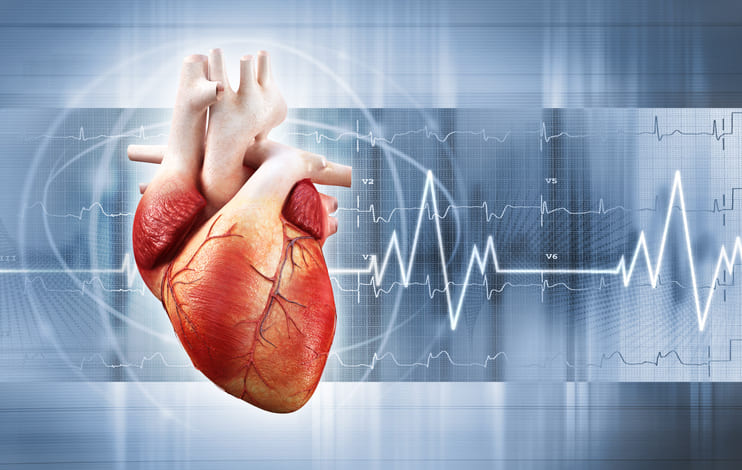