Few definitive guidelines exist for rigorous large-scale prospective evaluation of nonrandomized programs and policies that require longitudinal primary data collection. In Veterans Affairs (VA) we identified a need to understand the impact of a geriatrics primary care model (referred to as GeriPACT); however, randomization of patients to GeriPACT vs. a traditional PACT was not feasible because GeriPACT has been rolled out nationally, and the decision to transition from PACT to GeriPACT is made jointly by a patient and provider. We describe our study design used to evaluate the comparative effectiveness of GeriPACT compared to a traditional primary care model (referred to as PACT) on patient experience and quality of care metrics.
We used prospective matching to guide enrollment of GeriPACT-PACT patient dyads across 57 VA Medical Centers. First, we identified matches based an array of administratively derived characteristics using a combination of coarsened exact and distance function matching on 11 identified key variables that may function as confounders. Once a GeriPACT patient was enrolled, matched PACT patients were then contacted for recruitment using pre-assigned priority categories based on the distance function; if eligible and consented, patients were enrolled and followed with telephone surveys for 18 months.
We successfully enrolled 275 matched dyads in near real-time, with a median time of 7 days between enrolling a GeriPACT patient and a closely matched PACT patient. Standardized mean differences of < 0.2 among nearly all baseline variables indicates excellent baseline covariate balance. Exceptional balance on survey-collected baseline covariates not available at the time of matching suggests our procedure successfully controlled many known, but administratively unobserved, drivers of entrance to GeriPACT.
We present an important process to prospectively evaluate the effects of different treatments when randomization is infeasible and provide guidance to researchers who may be interested in implementing a similar approach. Rich matching variables from the pre-treatment period that reflect treatment assignment mechanisms create a high quality comparison group from which to recruit. This design harnesses the power of national administrative data coupled with collection of patient reported outcomes, enabling rigorous evaluation of non-randomized programs or policies.
© 2021. The Author(s).
Evaluation of a geriatrics primary care model using prospective matching to guide enrollment.
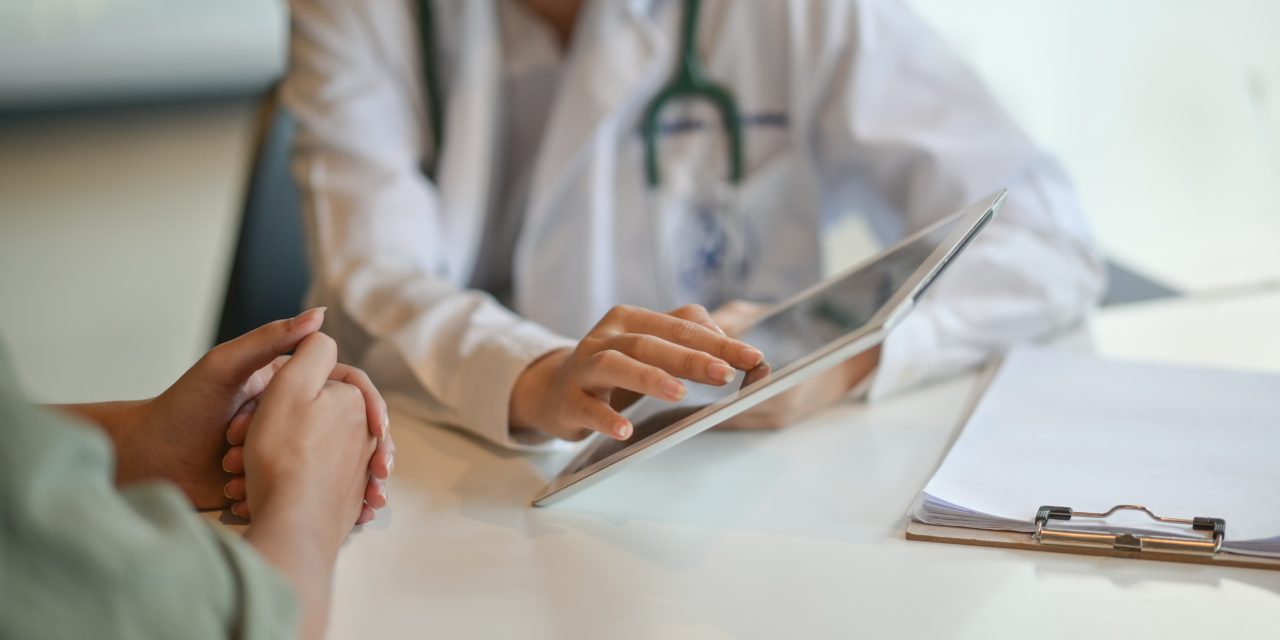