Endoscopy is essential for disease assessment in ulcerative colitis (UC), but subjectivity threatens accuracy and precision. We aimed to pilot a fully-automated video analysis system for endoscopic disease grading in UC.
A developmental set of high-resolution UC endoscopic videos were assigned Mayo endoscopic scores (MES) provided by 2 experienced reviewers. Video still image stacks were annotated for image quality (informativeness) and MES. Models to predict still image informativeness and disease severity were trained using convolutional neural networks. A template matching grid search was used to estimate whole-video MES provided by human reviewers using predicted still image MES proportions. The automated whole-video MES workflow was tested using unaltered endoscopic videos from a multicenter UC clinical trial.
The developmental high-resolution and testing multicenter clinical trial sets contained 51 and 264 videos, respectively. The still image informative classifier had excellent performance with a sensitivity of 0.902 and specificity of 0.870. In high-resolution videos, fully automated methods correctly predicted MES in 78% (41/50, κ=0.84) of videos. In external clinical trial videos, reviewers agreed on MES in 82.8% (140/169) of videos (κ=0.78). Automated and central reviewer scoring agreement occurred in 57.1% of videos (κ=0.59), but improved to 69.5% (107/169) when accounting for reviewer disagreement. Automated MES grading of clinical trial videos (often low resolution) correctly distinguished remission (MES 0,1) versus active disease (MES 2,3) in 83.7% (221/264) of videos.
These early results support the potential for artificial intelligence to provide endoscopic disease grading in UC that approximates the scoring of experienced reviewers.
Copyright © 2020 American Society for Gastrointestinal Endoscopy. Published by Elsevier Inc. All rights reserved.
Fully automated endoscopic disease activity assessment in ulcerative colitis.
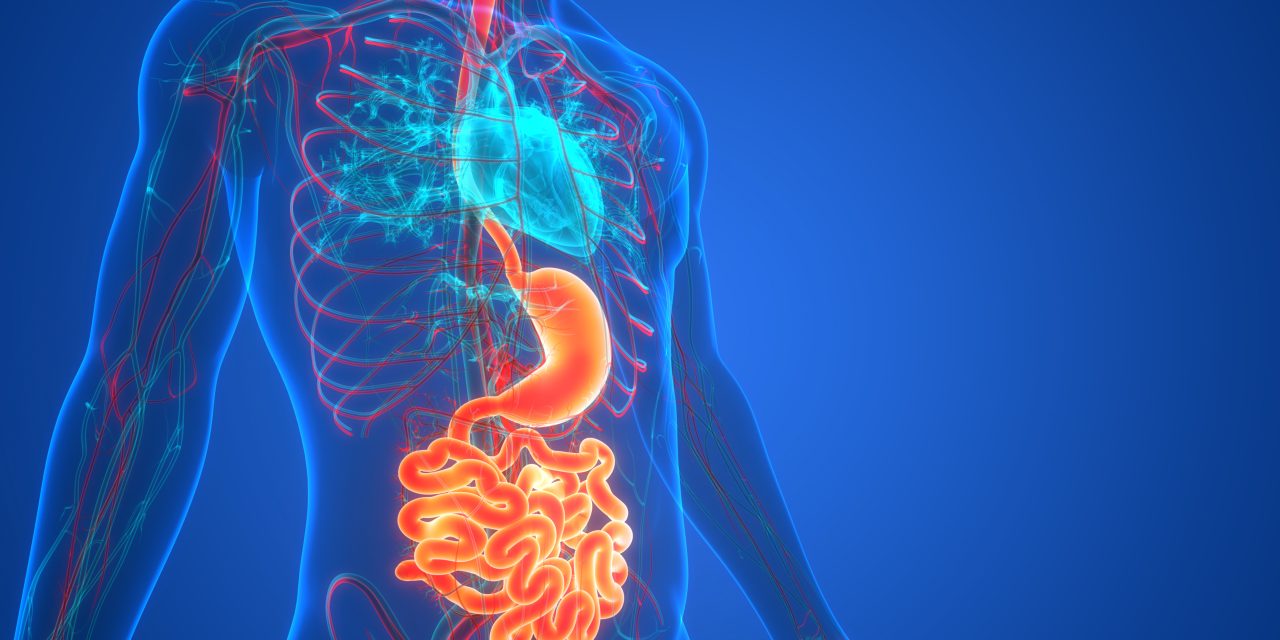