Over the past decade, there has been a groundswell of research interest in computer-based methods for objectively quantifying fibrotic lung disease on high resolution CT of the chest. In the past 5 years, the arrival of deep learning-based image analysis has created exciting new opportunities for enhancing the understanding of, and the ability to interpret, fibrotic lung disease on CT. Specific unsolved problems for which computer-based imaging analysis might provide solutions include the development of reliable methods for assisting with diagnosis, detecting early disease, and predicting disease behaviour using baseline imaging data. However, to harness this technology, technical and societal challenges must be overcome. Large CT datasets will be needed to power the training of deep learning algorithms. Open science research and collaboration between academia and industry must be encouraged. Prospective clinical utility studies will be needed to test computer algorithm performance in real-world clinical settings and demonstrate patient benefit over current best practice. Finally, ethical standards, which ensure patient confidentiality and mitigate against biases in training datasets, that can be encoded in machine-learning systems will be needed as well as bespoke data governance and accountability frameworks to encourage buy-in from health-care professionals, patients, and the public.Copyright © 2020 Elsevier Ltd. All rights reserved.
Imaging research in fibrotic lung disease; applying deep learning to unsolved problems.
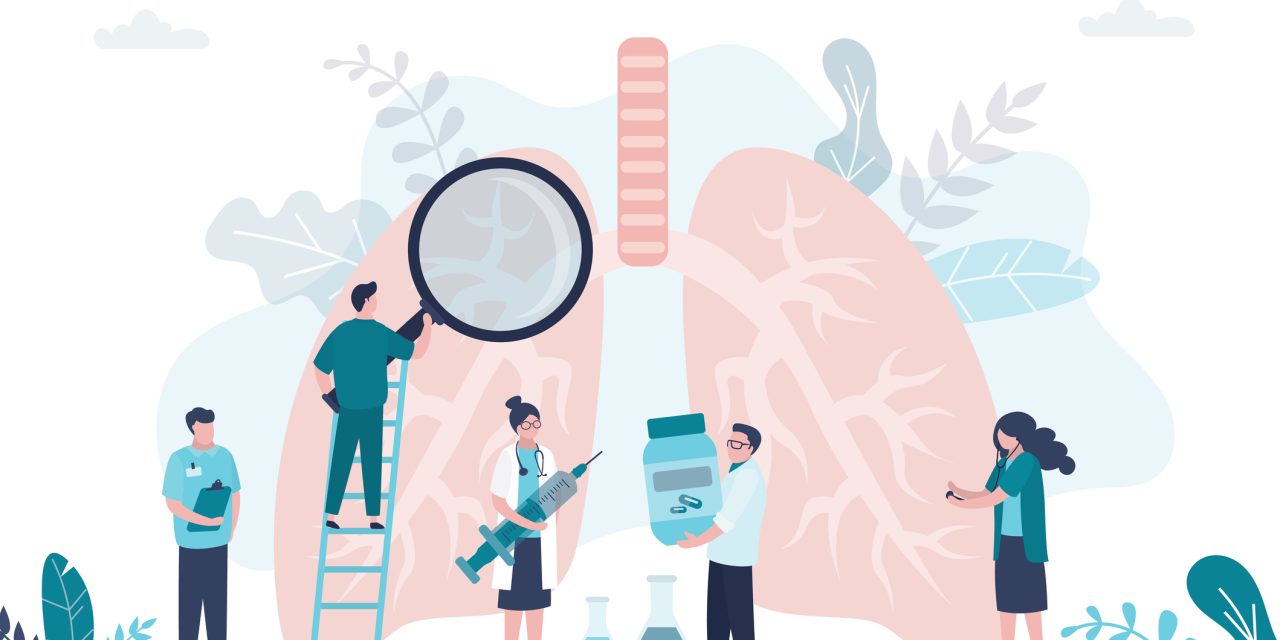