Gaining insights that cannot be obtained from health care databases from patients has become an important topic in pharmacovigilance.
Our objective was to demonstrate a use case, in which patient-generated data were incorporated in pharmacovigilance, to understand the epidemiology and burden of illness in Japanese patients with systemic lupus erythematosus.
We used data on systemic lupus erythematosus, an autoimmune disease that substantially impairs quality of life, from 2 independent data sets. To understand the disease’s epidemiology, we analyzed a Japanese health insurance claims database. To understand the disease’s burden, we analyzed text data collected from Japanese disease blogs (tōbyōki) written by patients with systemic lupus erythematosus. Natural language processing was applied to these texts to identify frequent patient-level complaints, and term frequency-inverse document frequency was used to explore patient burden during treatment. We explored health-related quality of life based on patient descriptions.
We analyzed data from 4694 and 635 patients with systemic lupus erythematosus in the health insurance claims database and tōbyōki blogs, respectively. Based on health insurance claims data, the prevalence of systemic lupus erythematosus is 107.70 per 100,000 persons. Tōbyōki text data analysis showed that pain-related words (eg, pain, severe pain, arthralgia) became more important after starting treatment. We also found an increase in patients’ references to mobility and self-care over time, which indicated increased attention to physical disability due to disease progression.
A classical medical database represents only a part of a patient’s entire treatment experience, and analysis using solely such a database cannot represent patient-level symptoms or patient concerns about treatments. This study showed that analysis of tōbyōki blogs can provide added information on patient-level details, advancing patient-centric pharmacovigilance.
©Shinichi Matsuda, Takumi Ohtomo, Shiho Tomizawa, Yuki Miyano, Miwako Mogi, Hiroshi Kuriki, Terumi Nakayama, Shinichi Watanabe. Originally published in JMIR Public Health and Surveillance (https://publichealth.jmir.org), 29.06.2021.
Incorporating Unstructured Patient Narratives and Health Insurance Claims Data in Pharmacovigilance: Natural Language Processing Analysis of Patient-Generated Texts About Systemic Lupus Erythematosus.
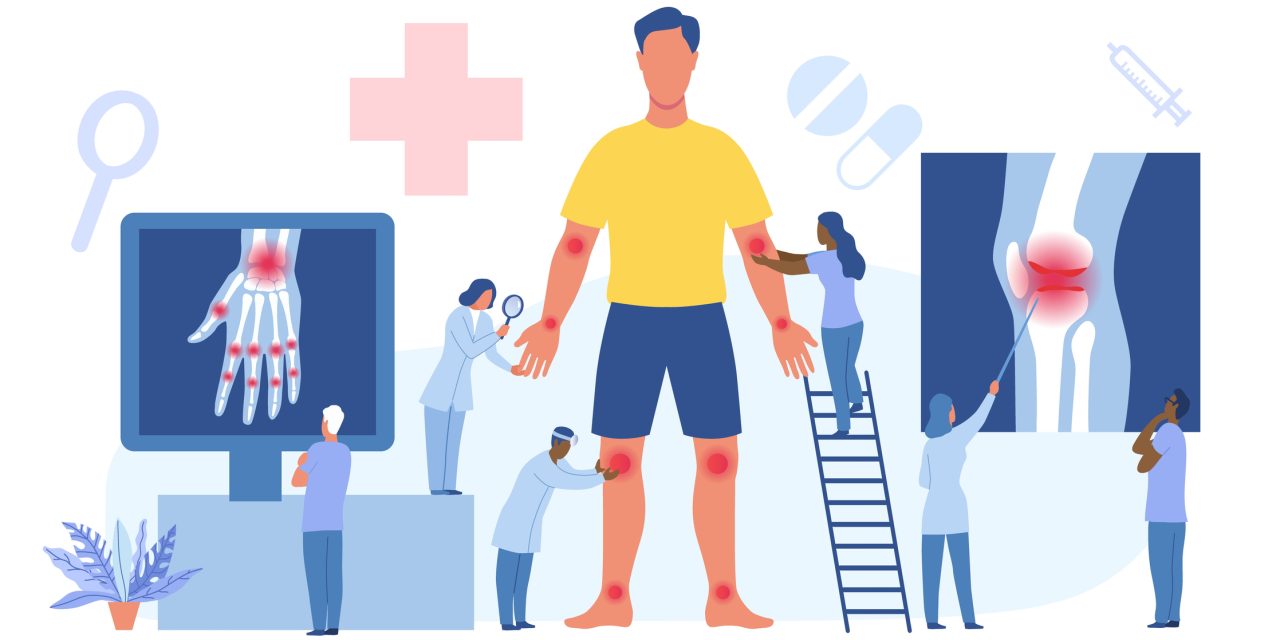