A downside of Deep Brain Stimulation (DBS) for Parkinson’s Disease (PD) is that cognitive function may deteriorate postoperatively. Electroencephalography (EEG) was explored as biomarker of cognition using a Machine Learning (ML) pipeline.
A fully automated ML pipeline was applied to 112 PD patients, taking EEG time-series as input and predicted class-labels as output. The most extreme cognitive scores were selected for class differentiation, i.e. best vs. worst cognitive performance (n = 20 per group). 16,674 features were extracted per patient; feature-selection was performed using a Boruta algorithm. A random forest classifier was modelled; 10-fold cross-validation with Bayesian optimization was performed to ensure generalizability. The predicted class-probabilities of the entire cohort were compared to actual cognitive performance.
Both groups were differentiated with a mean accuracy of 0.92; using only occipital peak frequency yielded an accuracy of 0.67. Class-probabilities and actual cognitive performance were negatively linearly correlated (β = -0.23 (95% confidence interval (-0.29, -0.18))).
Particularly high accuracies were achieved using a compound of automatically extracted EEG biomarkers to classify PD patients according to cognition, rather than a single spectral EEG feature.
Automated EEG assessment may have utility for cognitive profiling of PD patients during the DBS screening.
Machine learning for automated EEG-based biomarkers of cognitive impairment during Deep Brain Stimulation screening in patients with Parkinson’s Disease.
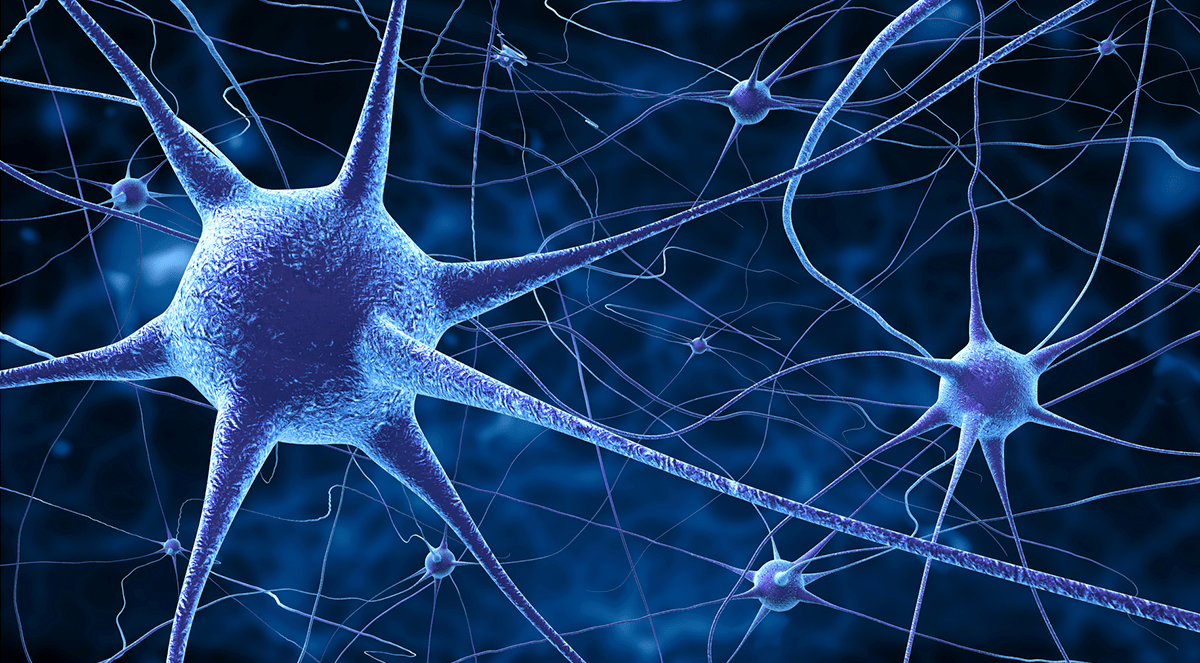