Psychiatric diagnosis and treatment have historically taken a symptom-based approach, with less attention on identifying underlying symptom-producing mechanisms. Recent efforts have illuminated the extent to which different underlying circuitry can produce phenotypically similar symptomatology (e.g. psychosis in bipolar disorder vs schizophrenia). Computational modelling makes it possible to identify and mathematically differentiate behaviorally-unobservable, specific reinforcement-learning (RL) differences in schizophrenia (SZ) patients versus other disorders, likely due to a higher reliance on prediction-error(PE)-driven learning associated with basal ganglia, and under-reliance on explicit value representations associated with OFC.
We use a well-established probabilistic-RL task to replicate those findings in individuals with schizophrenia both on (N=120) and off (N=44) anti-psychotic medications, and include a patient comparison group of bipolar patients with psychosis (N=60) and healthy controls (n=72).
Using accuracy, there was a main effect of group (F(3,279)=7.87, p<0.001, such that all patients groups were less accurate than controls. Using computationally derived parameters, both medicated and unmediated individuals with SZ, but not bipolar patients, demonstrated a reduced "mixing" parameter (F(3,295)=13.91,p<0.001), indicating less dependence on learning explicit value representations, as well as greater learning decay between training and test (F(1,289)=12.81, p<0.001). Unmedicated SZ also showed greater decision noise (F(3,295)=2.67, p=0.04).
Both medicated and unmedicated patients show overreliance on PE-driven learning, as well as significantly higher noise and value-related memory decay, compared to the healthy controls and the bipolar patients. Additionally, the computational model parameters capturing these processes can significantly improve patient/control classification, potentially providing useful diagnosis insight.
Copyright © 2021. Published by Elsevier Inc.
Using Computational Modelling to Capture Schizophrenia-Specific Reinforcement Learning Differences and Their Implications on Patient Classification.
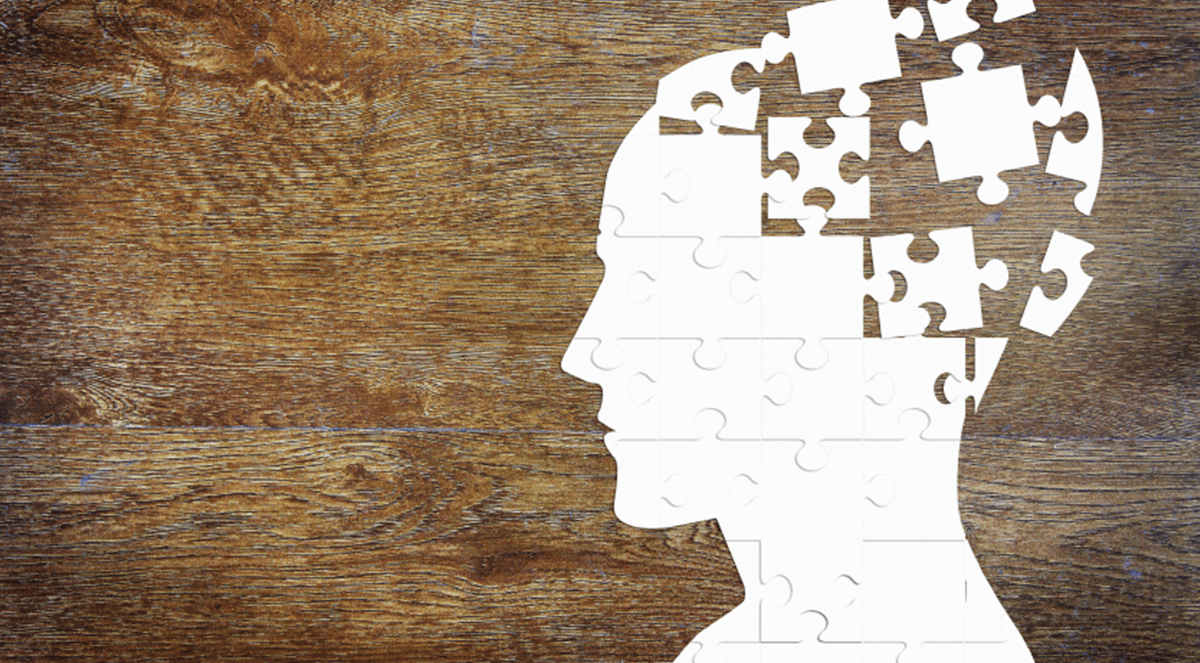