Vitiligo is a typical pigmented skin disorder, which affects up to 1% of the global population, and the appearance of the patient is severely affected. Its lesions are often characterized by large affected areas, irregular shapes, low contrast, and the difficulty of identifying under natural light. This paper proposes a weakly supervised vitiligo segmentation framework using only image-level annotation to perform segmentation. We first roughly locate the lesion region through the class activation map – the byproduct of a CNN-based classification model. By further exploring the class activation map and leveraging the local information of the image, we perform saliency propagation to produce segmentation with accurate boundaries and strong interpretability. Moreover, we collect a vitiligo image dataset named Vit2019, which contains 2000 images; to our best knowledge, this is so far the largest image dataset for vitiligo. The experiment shows our method not only achieves good results on Vit2019 with IoU of 72.7%, but also on the ISIC-2017 dataset, which contains other types of pigmented skin diseases (e.g., nevus, melanoma and seborrheic keratoses), our method achieves IoU of 54.2%. Our experiments demonstrate the superiority of the proposed model over the state-of-the-art.Copyright © 2020 Elsevier Ltd. All rights reserved.
VitSeg: Weakly supervised vitiligo segmentation in skin image.
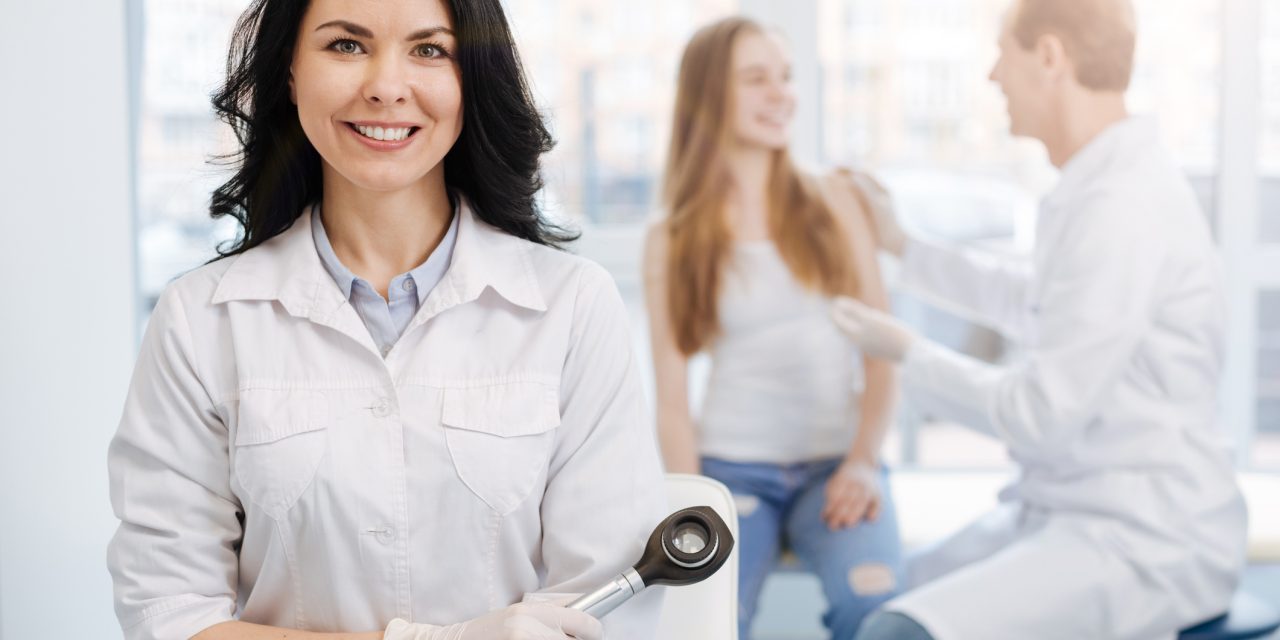